How data observability can boost IT performance in the banking sector
The finance sector has to move fast to keep up with technology. Read on to see how S2E helped Cedacri with data science and engineering.
Many industries have found themselves having to move quickly to keep up with changing technologies, and the world of fintech has felt this change more than most. Few consumer services command the desire for security that financial institutions such as banks do, while at the same time, customers expect the conveniences that come with instantly available information and services.
The general shift in all walks of business towards Software as a Service (SaaS) is one that makes economical sense in many cases. There is far more risk when developing cloud applications, making the cost-benefit analysis of having bespoke applications created much less appealing than it has been in the past. When the customers in question are banks, however, the stakes are raised even further.
Cedacri Group is a company that is tackling this seemingly insurmountable problem—offering IT services to fintech. Even a company considered to be the most important Italian company in this field needs help on occasion, and in this article, we’re going to look at how S2E (Solutions2Enterprises) provided that help in the form of tailored data management and engineering services.
Data Science and Engineering in The Banking Sector
As the world moves ever more into digital spaces, the science of data is becoming increasingly important. The science—perhaps even the art—of analysing data is one that can help to avoid accidents, prevent fraud, make remarkably accurate predictions on future trends, and more.
The reality of modern business is that companies have access to more data than they could ever have collected in the past. Such a wealth of information is a gold mine for organisations who are looking to make accurate predictions, determine where things aren’t working (and where they are) and more. Paradoxically, the problem with having all this data is that it can be overwhelming, and without an effective approach to analysing the data, it is essentially useless. That is where data engineering comes in.
Key Concepts Of Data Science In The Banking Sector
Here are some of the ways in which data science is enabling fintech to keep up with the immense pace of modern technology.
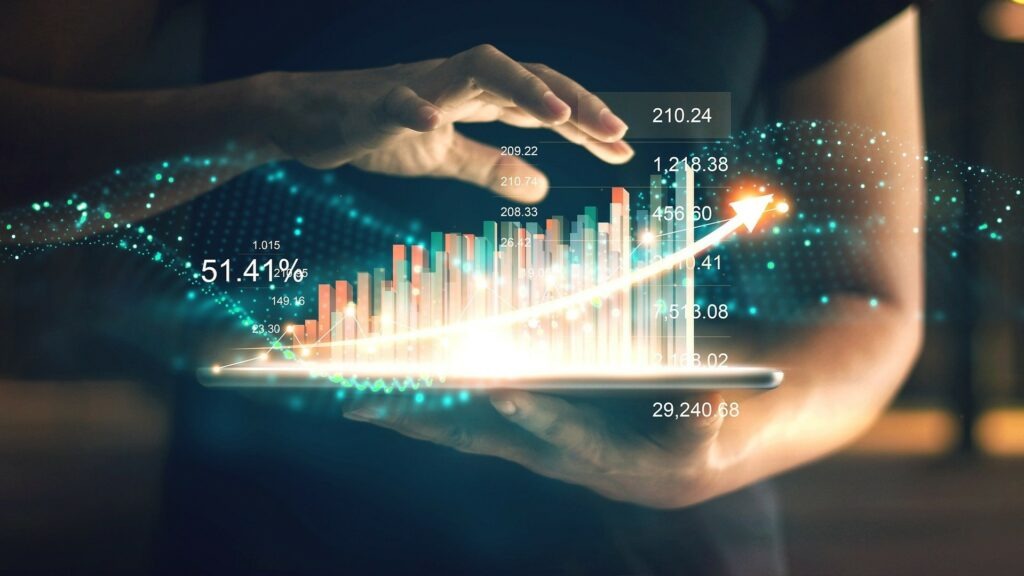
Managing Customer Data
The collection of customer data is something of a sore subject these days, with consumer sentiment increasingly shifting towards less data being better. Banks, however, are obliged to collect huge amounts of data in order to provide the services they do. These include things like advanced security features, credit services, and more.
Another key aspect to take into account is that customer data needs to be handled by companies and institutions in full compliance with the different international security standards such as GDPR (EU’s data security and privacy law).
The advent of digital banking has not only increased the amount of data that needs to be held on each customer, but it has also increased the opportunity to collect data. Data science is the mechanism by which banks can isolate what information is relevant. From there, data engineering can be used to identify trends, unlock potential revenue sources, and inform future business decisions.
Observability
Data observability was, in the past, the sole responsibility of software engineers.
Today, Observability involves various stakeholders because it allows organizations to delve into what their systems, services, and apps are really doing.
Observability means collecting KPIs in a non-invasive way of IT services. Considering that a performing IT is necessary for a performing business, observability is the way to measure and definitively improve the business of the organization.
Customer Services
Understanding the customer through data is essential for three main different purposes that involve the marketing, segmentation, and user experience spheres:
Personalised Marketing: Perhaps one of the most well-known uses of data science and engineering these days is to create a more personalised experience for consumers when it comes to the advertisements they see and the offers they are given. A deep understanding of personal data points enables banks and other financial organisations to make offers that are relevant to their customers in ways that the bank would never previously have known about.
Customer Segmentation Being able to single out groups of customers based on things like their spending habits, income, age, and a host of other data points enables financial institutions to make far-reaching decisions. These decisions can be made with more agility than would have been possible if every customer was considered individually, but with more accuracy than considering all the customers together with no segmentation.
Customer Service: Ultimately, banks provide a service, albeit a very specific one. Any public-facing service comes with the need for customer support, and eliminating the need for that customer support is a win-win situation for financial institutions. This is because customers are generally having a much better experience when they don’t need customer support, so reducing the need for it not only reduces costs in terms of employees offering support, it also means customer experience is being inherently improved as a result.
Data engineering not only makes it easier to identify areas that need work to make the customer experience more intuitive but also makes it easier to automate parts of the customer support experience.
Actionable Analytics in Real-Time
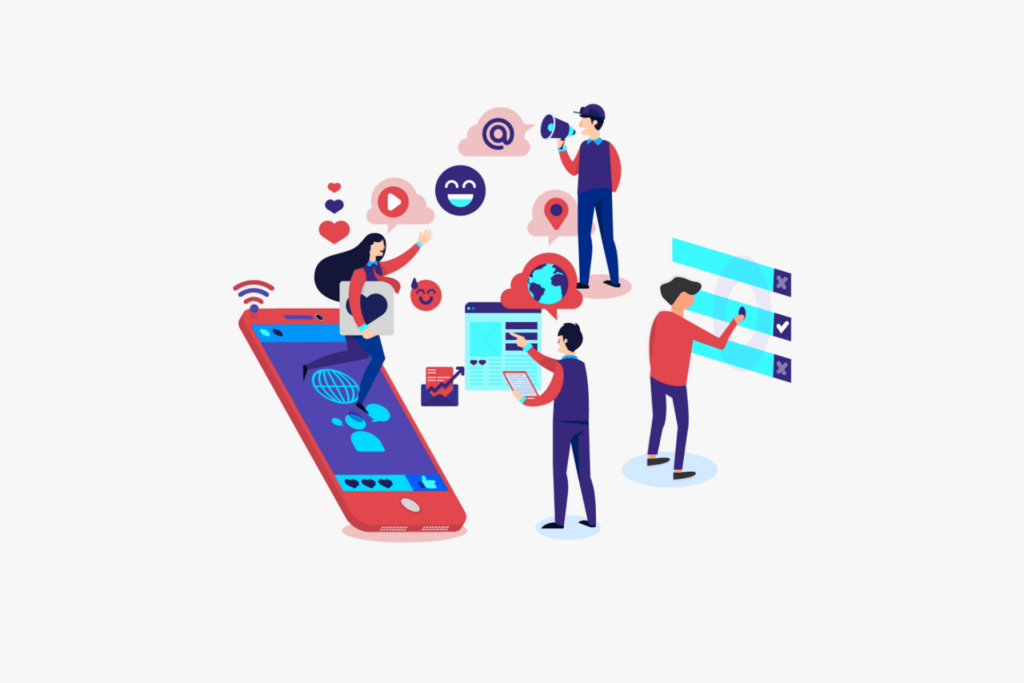
Being able to analyse data in real-time enables large organisations like banks to be more agile, whether it’s responding quickly to what the data says, or taking proactive measures based on predictions.
Data streaming is an innovative architecture that is now accepted as “real-time”: data is processed as it moves from source to destination, so the data value is immediately extracted making it available instantly to decision-makers. These decision-makers can also be intelligent agents, so not only analysis but also actions become real-time.
Other Areas
This is by no means a definitive list of the ways in which data engineering and engineering can help financial institutions improve their service and become more efficient and agile operations. Other examples include;
- Predicting the lifetime value of a customer
- Modelling investment risk
- Making recommendations to customers, such as in times of financial hardship
- Fraud detection through knowledge of customer habits
The S2E Case Study
Cedacri specialises in integrated IT services for the banking sector, essentially providing banks with the tools they need to capitalise on the data available to them while removing the cost of investing in the necessary systems. In bringing in S2E to consult on their implementation of the Elastic platform, Cedacri—whose customers are banks and other financial institutions—has been able to capitalise on the key aspects of data science and engineering. The Elastic platform collects the logs of all systems from the IT architectures of their client banks, the analysis of which allows for the measurement of key performance indicators (KPIs), highlighting areas where systems may not be working optimally.
From monitoring data silos to delivering hardware infrastructure metrics, the needs of Cedacri were met with solutions such as providing a single view of performances for the entire data ecosystem. Presenting this overview in a simple, digestible form allows for a reactive approach to problems as they develop.
These systems bring the real-time availability of KPIs to the screens of relevant parties in a user-friendly manner, reducing the need for additional training for Cedacri employees. The system is non-intrusive and safe and allows for a range of alerts, as well as a predictive approach to business decision-making.
Conclusions And What’s Coming
It has long been known that a large portion of customer support matters are to do with relatively simple problems that could be avoided with more readily available information or simple options to find the things the customer is looking for. A common example of this is providing an automated way for a customer to hear their bank balance before putting them through to a human support agent.
As fintech progress, automated resolutions are going to be possible for an increasing number of issues, both reducing the cost of providing customer support, and improving the experience of the customers who need that support. The intelligent use of data science and engineering should also see more ways to reduce the need for customer support to come into play.
Fonte: Codemotion